The field has been around for almost a century, with research dating back to the 1930s on caloric restriction in mice. Despite all, we currently have zero FDA-approved interventions for aging or longevity.
The current decade is the critical decade.
Over the last ten years, the field has cleared some critical obstacles for a regulatory pathway. In 2015, a group of leading academics in the field met with the FDA about the TAME (Targeting Aging with Metformin) Trial and indicated the FDA agreed with the plan. In 2023, Loyal received a historic FDA milestone of reasonable expectation of effectiveness for lifespan extension in large dogs. And recently, BioAge announced a phase 2 clinical trial with Eli Lilly on a drug combination (azelaprag & tirzepatide) for musculoskeletal protection alongside GLP-1s.
Over the next ten years, we will see more therapeutics, tools, and technologies in the field move from lab to clinic than ever before. The longevity funnel framework (LFF) is how we think about translation:
To rigorously show that healthy lifespan extension in humans is possible, we still have significant strides to make in each “layer” of the funnel:
Mechanisms of aging
Development of a casual understanding of the mechanisms of aging, in relation to functional outcomes.
Interventions for aging
Design more interventions that target aging with creative, ambitious, and scientifically rigorous approaches (including small molecule approaches and beyond).
Improving the safety and efficacy of existing modalities and developing new ones suitable for long-term interventions that potentially require repeat dosage.
Measures and models of aging
Development of models of aging, especially naturally aged model organisms and non-model organisms.
Validation of methods to measure and quantify aging at molecular, cellular, tissue, and organism levels.
Consensus around aging endpoints for clinical studies and subsequently useful biomarkers.
Clear strategies and FDA regulatory pathways for cost-effective aging clinical trials in humans.
Mechanisms of aging
Connecting our understanding of aging at the molecular and cellular levels with functional outcomes.
As we age, disease incidence increases dramatically (healthcare costs also skyrocket)!
A cumulative loss of function and accumulation of damage leads to aging, which corresponds to a drop in survival probability on the Kaplan-Meier curve. Healthspan and lifespan extension ideally mitigate this process. We do not want one without the other. Hence, the goal for more years in good health = healthy lifespan extension.
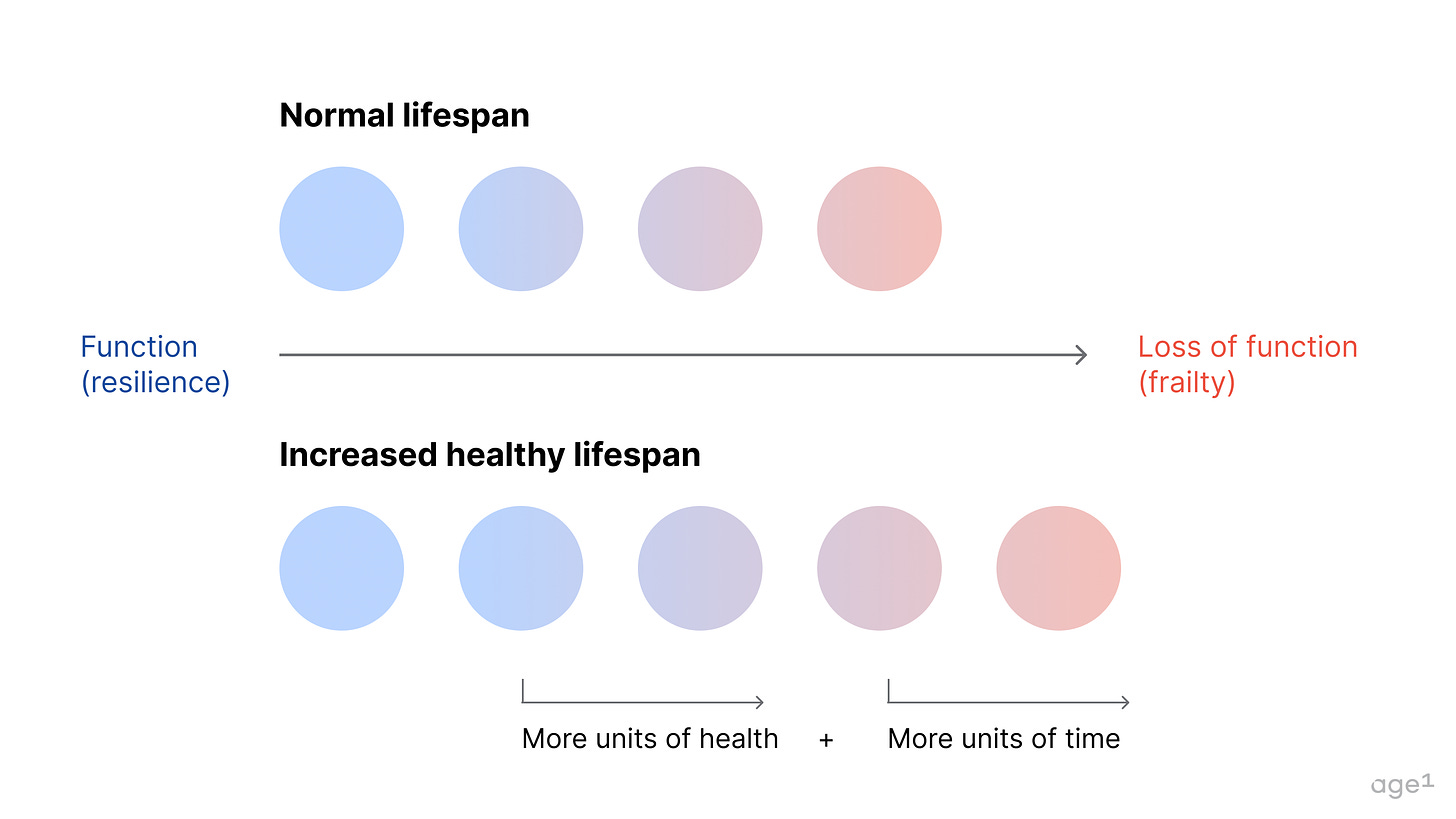
Aging is much more than a change in x over time. It is multimodal, multifactorial, and highly variable across space and time. The rate of aging varies across organs and throughout a lifetime. There are also sex-related differences, such as in immune system aging.
Approaching the question of “What drives aging?” using the hallmarks of aging is reductionist (credit is still due as it provides an overview of current areas of research!). The hallmarks do not capture how aging is a complex network of changes across space and time. As a result, we still have many gaps in understanding of how pathways implicated in aging drive outcomes at the organism level.
The age-old question of genotype to phenotype remains open. Although difficult, we have not yet established a quantitative and causal understanding of the mechanisms of aging. Targeting the drivers of aging is the ultimate goal of designing a therapeutic, so understanding the causality of mechanisms is critical. Regardless of what “theory of aging” these mechanisms are derived from (ex: metabolic regulation of aging, information theory of aging), the physiological outcomes are what matter. These mechanisms should be connected to function, feeling, and survival for clinical relevance.
If you think about aging as some process that maps causal mechanisms to phenotypes, a therapeutic targeting of these mechanisms is unlikely one-to-one or one-to-all in its effect. There is no one, all-encompassing “magical” pathway that modulates all aging phenotypes. Modulating sets of mechanisms correspond to a target-based approach whereas modulating sets of aging phenotypes correspond to a phenotype-based approach. To avoid exploring an infinite solution space, we need to account for both to varying degrees.
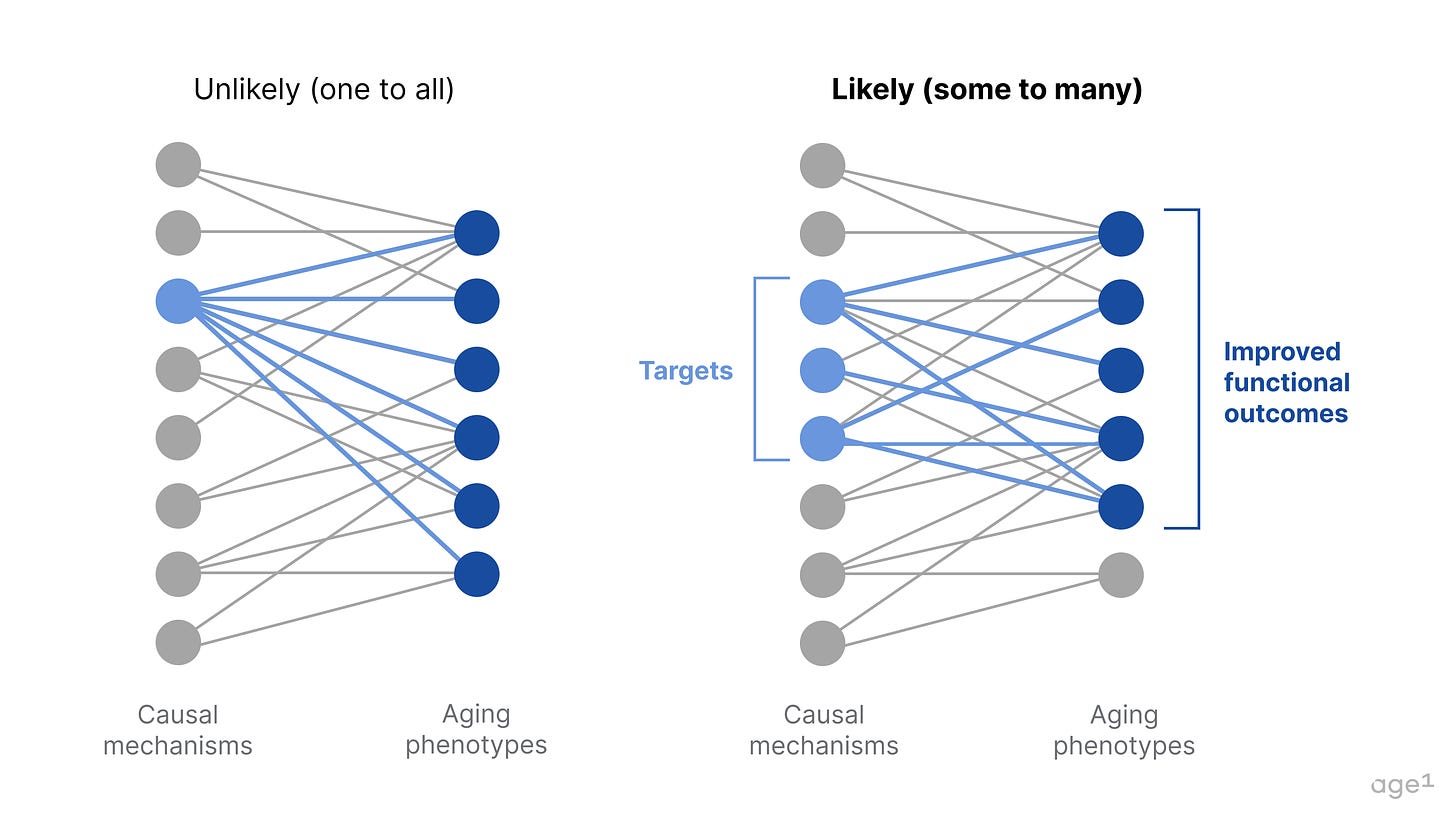
Relationships between mechanisms form complex networks. The same network structure applies to aging phenotypes at cellular, tissue, or organism levels. These networks then interact via biological pathways to produce systems-level, functional outcomes (ex: some of these interactions as a map). With aging, the interactions within and between these networks also change over time.
Besides reducing the solution space, this is relevant because approaching from a systems biology perspective might lead to therapeutic approaches not considered before. Modulating one system could be the “fix” for another system’s age-related disease (ex: regulating cardiovascular systems’ effects on neurodegenerative diseases via targeting neurovascular coupling).
Then, from a complex systems perspective, we can consider how these systems self-regulate for homeostasis. Aging leads to a breakdown of feedback loops over time, as systems lose their ability to maintain balance. This is even more challenging to model but could be a way forward to discern drivers of aging versus factors that are simply involved via related pathways/etc.
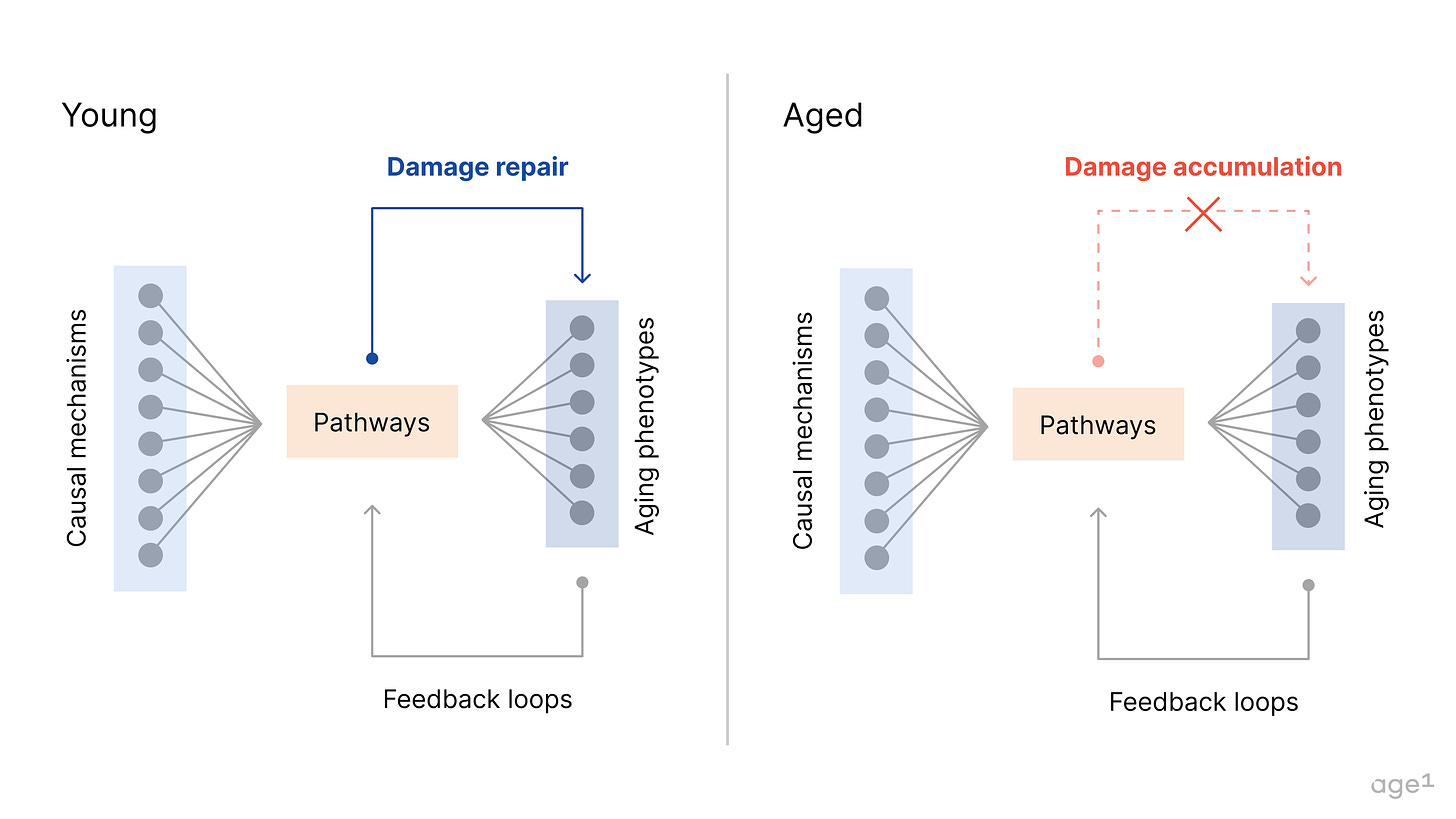
For more on the biology of aging, here are some resources:
Historical perspective: a review of progress to date from Campisi et. al
Hallmarks perspective: the 2023 hallmarks of aging from López-Otín et. al (2013 version)
Overview: the original longevity FAQ by Laura Deming, an extended longevity FAQ by José Luis Ricón
Textbooks: Handbook of the Biology of Aging (covers some systems-level aging) and Systems Medicine: Physiological Circuits and the Dynamics of Disease, by Uri Alon (for systems-level modeling with some math)
Overall, capturing the networked nature of changes in aging will help improve the translatability of what we study. For computational approaches, this nuance will guide more meaningful inference-based learning. For tool development, studying aging across the biological scale will help answer more causality-related questions. Finally, for therapeutic interventions, a casual understanding of aging means more efficacious therapeutics that target drivers of aging. We’ll have better indication and target selection, a clear set of phenotypes to screen for, and an improved ability to customize the modality based on the mechanism of action. However, an incomplete picture does not impede our ability to move forward: we can design interventions with what we rigorously understand so far.
Interventions for aging
Broader consideration of possible interventions for aging.
Small molecule drugs alone, single or combinatorial, are not medicine’s endgame. Exploring more alternative approaches is essential for addressing the steep decline in pharma R&D efficiency. The future entails vastly more ambitious scientific moonshots (but equally as rigorous as ambitious).
As a 1st gen approach, small molecules will likely be the first way to target system-level dysfunction and delay aging in humans. Since initial proof of lifespan extension in mice and rats via caloric restriction, multiple pioneering studies have found ways to alter organismal aging using genetic interventions (ex: via modifying age-1 and daf-2 genes in C.elegans) and small molecules (ex: rapamycin and metformin in mice via NIH’s Interventions Testing Program). But if we are to improve the clinical efficacy in which we can target aging, we’ll need to pursue 2nd gen approaches that replace, restore, or pause aging.
2nd gen approaches might sound like sci-fi, but most have deep historical roots and are widely accepted in different contexts. Tissue replacement originated from bone autografts and skin allografts date back to 1668. For whole organ replacement, the first successful kidney transplant was performed in 1954 by Dr. Joseph E. Murray, who later received a shared Nobel Prize for Medicine in 1990. Similarly, regenerative medicine was built on the discovery of transplantable blood stem cells in 1961 by James Till and Ernest McCulloch. Reprogramming has its roots in John Gurden’s work from 1962, where he discovered that the specialization of cells is reversible. Cryobiology dates back even further, to 1665 with Robert Boyle’s studies on the effects of freezing temperatures on living animals. And today, freezing oocytes and sperm is standard practice in reproductive health.
From this historical perspective, the novelty in 2nd gen approaches will mostly come from developing technical maturity in these science areas and demonstrating clinical utility for aging or longevity. These interventions are (and should be) grounded in decades, if not centuries, of foundational scientific work.
Categories of interventions for aging
Delay (delay or prevent aging): most existing approaches fall within this category, which are hallmarks of aging-esque.
Replace (replace aged, damaged cells and tissues with youthful ones): possible approaches include cell therapy, mechanical parts, and tissue/organ transplantation (supplied by xenotransplantation, growing human organs ex vivo, 3D bioprinting, etc). Replacing and restoring aging are not mutually exclusive (ex: the replacement theory of aging as an approach to enhance neural plasticity and unlock neurogenesis).
Restore (turn the clock back to restore the age of cells and tissues to a youthful stage): possible approaches include full or partial cellular reprogramming using transcription factors, though more studies are required to validate that “reversal” of age has occurred through such mechanisms without inducing dedifferentiation to harmful levels and extensive, subsequent teratoma formation. Broadly, most approaches within regenerative medicine could be categorized as possible ways to restore aging.
Pause (pause the aging clock in human cells and tissues by inducing stasis): possible approaches include torpor, hibernation, and cryopreservation at the cellular, tissue/organ, and organism levels. This is especially key for enabling longer-term storage of organs before transplantation without significant tissue damage.
For more detailed categorization (non-exhaustive), here are some resources:
Longevity biotech landscape by Ada Nguyen
Synthesis of categories from the hallmarks of aging, 7 pillars of aging, and SENS damage types by Karl Pfleger
An up-to-date overview of bottlenecks and underlooked areas by Amaranth Foundation (not a categorization)
Comparing 1st gen vs 2nd gen approaches
In our view, there are three ways in which 1st and 2nd gen approaches differ (for more considerations at this stage of development, see Celine Halioua’s How to Build a Biotech):
Indications: the FDA has not (yet!) given official guidance on a possible regulatory path for an indication representing aging or a proxy of aging. In the interim, those for age-related diseases are most relevant. 1st gen approaches can take on greater regulatory risk to forge these new indications, as they require less innovation on modality. Loyal took the first step and has shown it is possible to obtain an indication for lifespan extension in large dogs. The logical next step is to obtain a similar indication for humans.
Modality: we are unlikely to design successful interventions that can replace, restore, or pause aging with well-established modalities like small molecules. To achieve functional improvements with a drastic effect on aging, these 2nd gen approaches will require innovation on emerging modalities with less technical maturity. Examples include cell and gene therapies, which need further development in safety and efficacy.
Possible effect size: 2nd gen approaches are more likely to achieve a greater effect size, which likely translates to increased healthy lifespan extension (effect size is the magnitude of difference between two groups, independent of the sample size). With a greater effect size, we also do not need such large samples to achieve statistical significance, which could help reduce clinical trial costs. When a drastic effect is observed, it will be obvious the therapeutic works without extensive statistical modeling (ex: Neurona’s regenerative cell therapy for epilepsy treatment where a significant effect was observed in the first 2 patients).
Regardless of differences, the parallel development of 1st gen and 2nd gen is crucial. Success in 1st gen acts as a proof point of real progress in the field, critical for attracting big pharma, institutional investors, and top talent. 1st gen approaches also pave the way for appropriate indications, so there is a path forward for pushing 2nd gen approaches to indications more closely relevant to aging.
Next, to test modalities and quantify effect size on the indication(s) of interest, we need good models and measures of aging.
Models & measures of aging
Creating robust models and measures of aging that are clinically relevant.
Many current models and measures of aging do not meet the bar for clinical translation. If a model and measure have low predictive validity, we are more likely to get a false positive or negative (bad!). We want good models and good measures of aging to increase the likelihood of a true positive.
False positive (type I errors): We may observe a desired effect size, but the intervention has limited or no clinical utility → Translation fails in phase I to III clinical trials (and $$ goes down the drain).
False negative (type II errors): We may not observe a desired effect size, but the intervention has some clinical utility → The desired effect size can be observed with alternative models/measures.
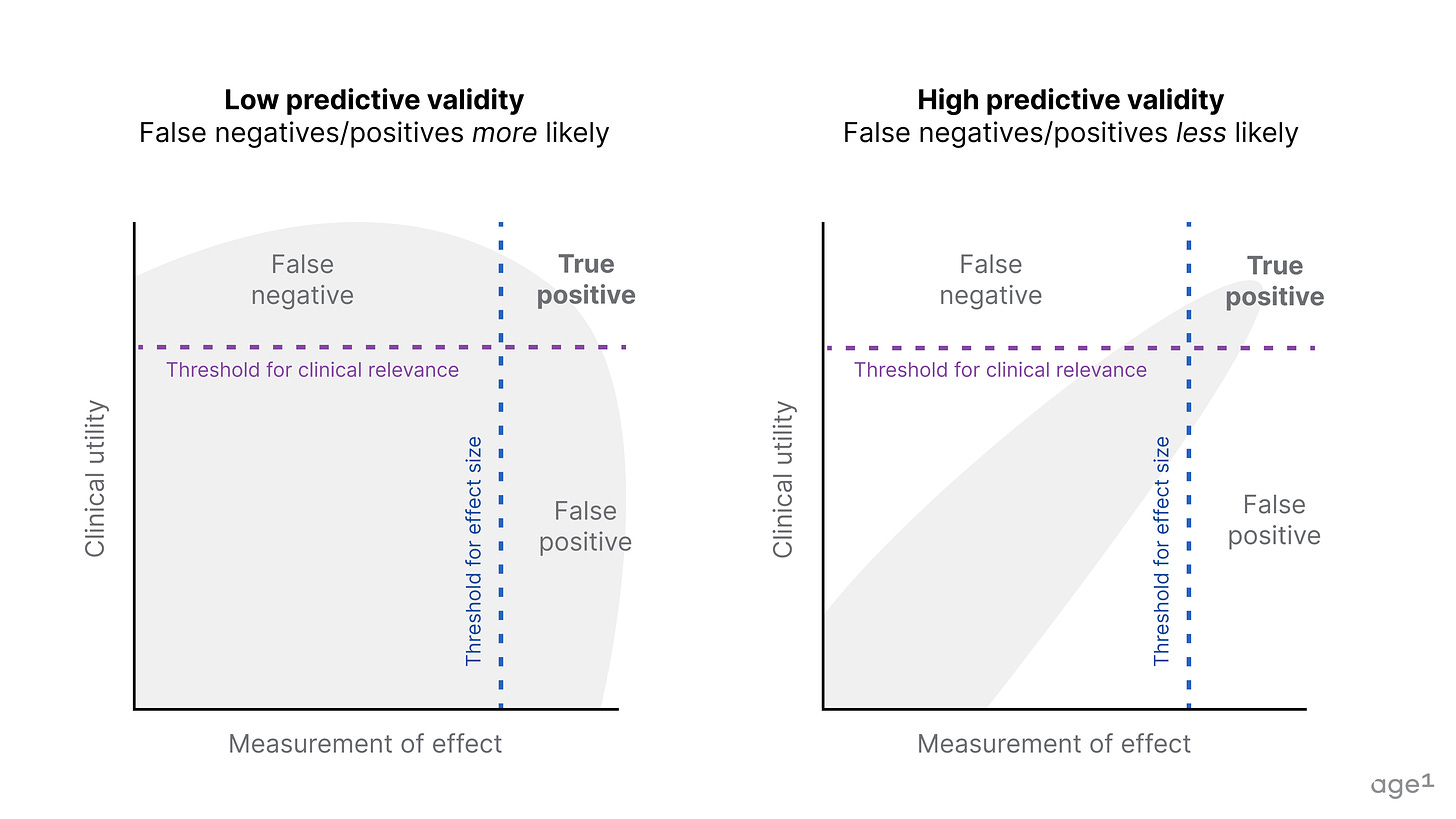
Disease models that don’t align with human disease outcomes and measurements via biomarkers with limited validation result in misguided candidate selection. This problem is universal to bio R&D, but aging adds the element of time to models and measurements, which complicates the design of experimental controls and appropriate statistical modeling (no p-hacking!).
NIH’s Interventions Testing Program (ITP) serves as a gold standard. ITP tests single drug interventions using genetically heterogenous mice (UM-HET3, four-strain cross), with replicates across three sites and statistical power of 80% for a 10% change in median lifespan extension. Despite the rigorous design, the ITP is limited in throughput and combinatorial testing.
Models of aging
Preclinical models span theoretical models (in silico), engineered models (in vitro/ex vivo), and model/non-model organisms (in vivo). A table of notable model and non-model organisms is in the appendix.
In silico and in vitro models can help drive faster iteration. One advantage is the ability to use human data and samples, which addresses a key limitation of in vivo models. However, the predictions are only as good as the data and the modeling as good as our understanding of biology. So, either we improve our models (which will take more time) or compensate with large volumes of data and massively parallel, high-throughput screening. To do so, we need more biobanks for human cell/tissue samples paired with de-identified and longitudinal genetic, lifestyle, and health data like the UK Biobank. In the long run, the development of microphysiological systems (ex: organ-on-a-chip systems) with physiological relevance for aging is also necessary, given the FDA Modernization Act 2.0.
In contrast, in vivo models can better capture the biology as we scale up to more complex organisms. However, no model organism compares to a full human, so we similarly need more high-throughput approaches to compensate. This includes low-cost production of genetically diverse and naturally-aged organisms, with automation where possible, biobanks for model and non-model organisms like the mouse gut microbial biobank, and more studies like the Zoonomia Project but focused on aging biology.
Above all, more models do not equate to better modeling. A single model with good predictive validity will always beat out an indefinite combination of bad models.
Measures of aging
There are endless possibilities of what to measure at the molecular, cellular, tissue/organ, or organism levels (ex: possible measurements for age-related diseases and emerging areas for molecular/cellular damage accumulation assays).
Beyond what metrics to measure, how the measure is correlated with aging is also critical. We want to capture state changes over time, such as shifts in survival curves, changes in composite scores, or fluctuations detectable by assays or aging clocks. For FDA interest, measures should capture function, feeling, and survival. These phenotypic outcomes are crucial for the design of clinical trial endpoints. A table of examples of molecular and non-molecular measures of aging is in the appendix.
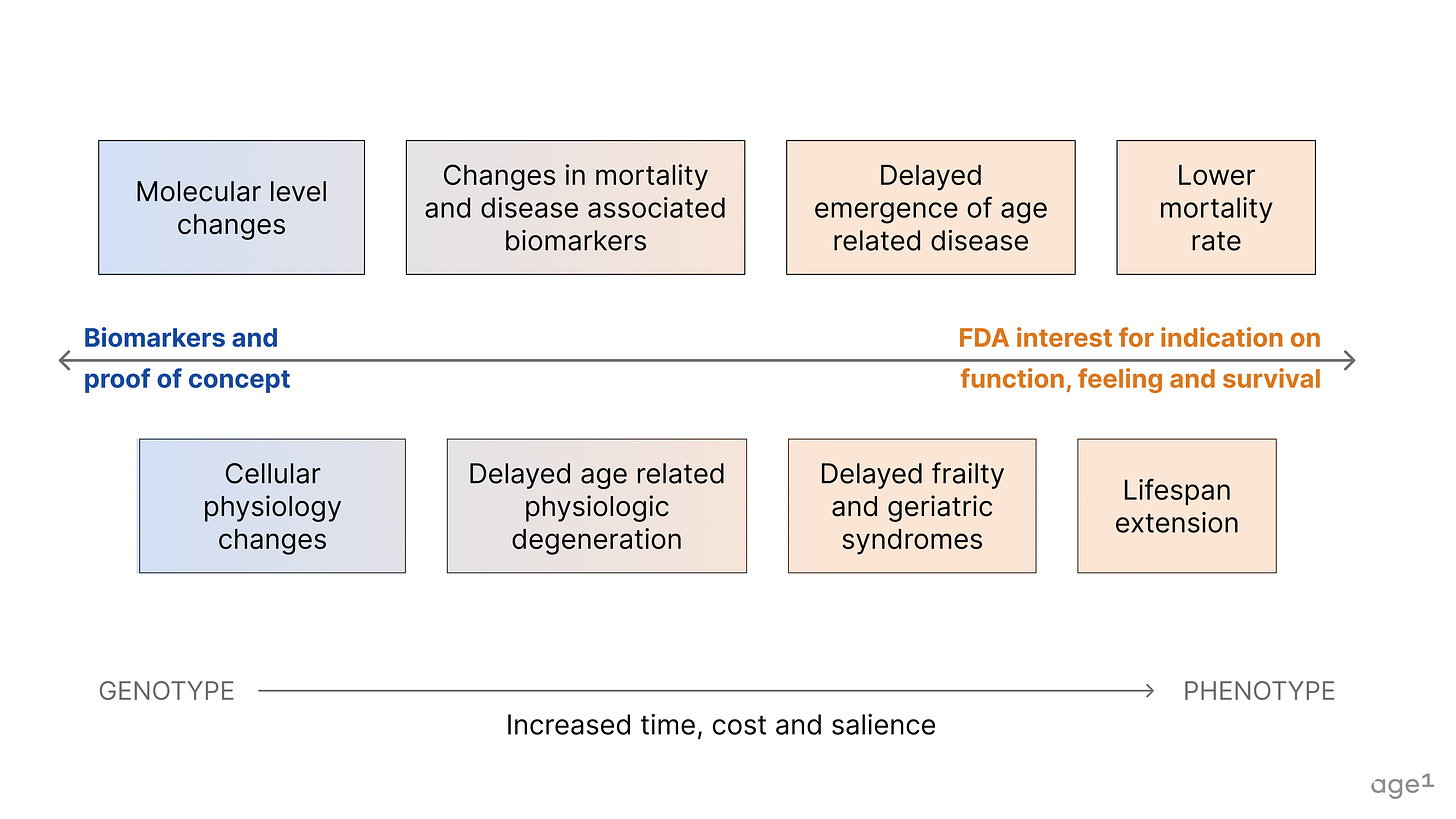
Endpoints serve as benchmarks to evaluate the relevance and reliability of biomarkers and proof of concept measures. A measure that accurately reflects the biology of aging needs to show a rigorous correlation with endpoints such as survival rates, disease progression, and symptom improvement.
Appendix
Table of model organisms
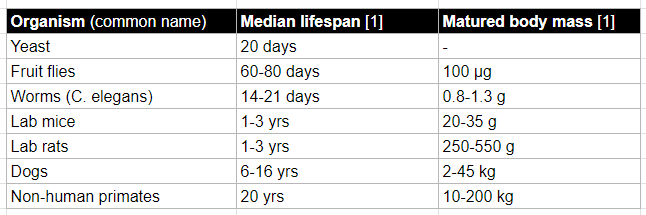
Table of non-model organisms (examples)
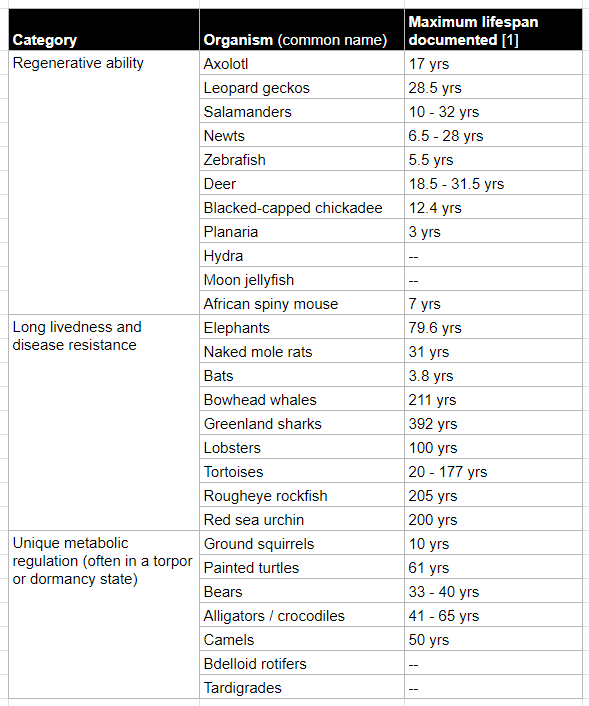
Table of measures of aging (examples)
Acknowledgements
All resources related to longevity/aging referenced in the piece or during the writing process are compiled here. The longevity funnel framework was largely inspired by the Amaranth Foundation’s “Bottlenecks of aging” and Celine Halioua’s “What the aging field needs”.
Special thank you to everyone on the age1 team: Alex Colville for extensive brainstorming support, Alan Tomusiak for inspiring V1 of the LFF, and Kat Kajderowicz, Alex Kesin, Carol Magalhaes, and Lily Clayton for helpful feedback.